In today’s data-driven world, organizations rely heavily on data to inform their decision-making processes. However, the effectiveness of these decisions hinges on the quality of the data being utilized. Data quality indicators serve as essential metrics that enable businesses to monitor and evaluate the integrity, accuracy, and reliability of their data. By implementing a strategic framework based on these indicators, organizations can ensure that they are using high-quality data in their operations, ultimately leading to improved performance and better outcomes.
Understanding Data Quality Indicators
Data quality indicators are specific metrics or measures that provide insight into various aspects of data quality. These indicators can encompass a range of attributes, including accuracy, completeness, consistency, timeliness, and relevance. For example, accuracy relates to how closely data reflects the real-world scenarios it represents, while completeness measures whether all necessary data points are available. By focusing on these indicators, businesses can gain a comprehensive understanding of their data’s quality and identify areas for improvement.
Importance of Data Quality in Business
High-quality data is critical for effective business operations. Poor data quality can lead to flawed analyses, misguided strategies, and ultimately, lost revenue. In contrast, high-quality data enhances decision-making processes, fosters trust among stakeholders, and improves overall organizational efficiency. By prioritizing data quality indicators, businesses can create a culture of accountability and continuous improvement, driving better decision-making at all levels.
Establishing a Strategic Framework
To effectively monitor and evaluate data quality, organizations must establish a strategic framework that aligns with their business goals. This framework should include the following components:
1. Define Data Quality Objectives: Organizations should begin by clearly defining their data quality objectives. These objectives should align with the overall business strategy and specific operational needs. For instance, if a company aims to improve customer satisfaction, it may prioritize data accuracy in customer feedback and service metrics.
2. Identify Key Data Quality Indicators: Once objectives are established, organizations need to identify the key data quality indicators that will help measure progress towards these goals. This involves selecting the most relevant metrics based on the defined objectives. For example, if completeness is a priority, organizations might track the percentage of required data fields that are filled in their databases.
3. Develop Data Quality Standards: Organizations should establish clear standards and definitions for each indicator. This ensures that everyone involved in data management understands what constitutes high-quality data. For instance, defining what “accuracy” means in the context of sales data may involve setting parameters around acceptable error rates.
4. Implement Data Quality Monitoring Processes: Regular monitoring of data quality indicators is essential for identifying issues and implementing corrective actions. Organizations should leverage automated tools and technologies to track these indicators in real-time. This proactive approach allows businesses to address data quality issues before they escalate into larger problems.
5. Foster a Data Quality Culture: Creating a culture that values data quality is vital for long-term success. Organizations should encourage employees to take ownership of data quality within their respective roles. Providing training and resources can help staff understand the importance of maintaining high data quality and the impact it has on business outcomes.
6. Review and Adjust: Finally, organizations must regularly review their data quality indicators and adjust their strategies as needed. This involves revisiting their objectives, assessing the effectiveness of their monitoring processes, and making necessary changes to the framework. Continuous improvement is key to ensuring that data quality remains a priority and evolves alongside changing business needs.
Challenges in Data Quality Management
Implementing a framework for monitoring and evaluating data quality is not without its challenges. Common obstacles include data silos, where information is isolated within different departments, leading to inconsistencies. Additionally, varying data standards across different teams can complicate the assessment of data quality. Organizations may also face resistance to change from employees who are accustomed to existing data management practices. Overcoming these challenges requires strong leadership, clear communication, and a commitment to fostering a data-driven culture.
Leveraging Technology for Data Quality Improvement
Technology plays a crucial role in enhancing data quality management. Advanced tools such as data cleansing software, data profiling tools, and data governance platforms can automate the monitoring of data quality indicators. These technologies not only streamline data management processes but also provide valuable insights through analytics. By harnessing the power of technology, organizations can more efficiently identify data quality issues and implement solutions.
The Role of Stakeholders in Data Quality Assurance
Collaboration among stakeholders is critical for effective data quality assurance. Involving various departments—such as IT, operations, marketing, and finance—in data quality initiatives ensures a holistic approach to data management. Stakeholders can provide insights into their specific data needs and help shape the framework to address those needs effectively. This collaborative effort fosters a sense of ownership and accountability, ultimately leading to improved data quality across the organization.
Operational Excellence
Implementing a strategic framework for data quality indicators is essential for effective business monitoring and evaluation. By focusing on defining objectives, identifying key indicators, establishing standards, and fostering a culture of data quality, organizations can enhance their decision-making processes and drive better business outcomes. Embracing technology and collaboration among stakeholders further strengthens this framework, ensuring that businesses remain agile and responsive in an increasingly data-driven world. Viewing data quality indicators not just as metrics but as integral components of a successful business strategy will pave the way for sustainable growth and operational excellence.
A Strategic Framework for Effective Business Monitoring and Evaluation
Share
Tweet
Email
Sponsored Post
This story is paid for by an advertiser. Members of the editorial and news staff of the Santa Clarita Valley Signal were not involved in the creation of this content.
Related To This Story
Latest NEWS
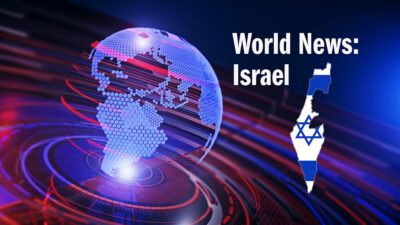
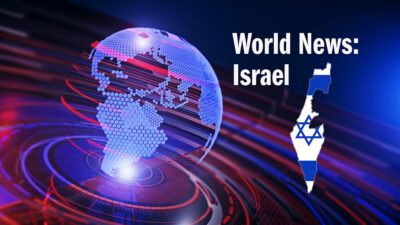
Trump calls for Gaza cease-fire deal
June 30, 2025
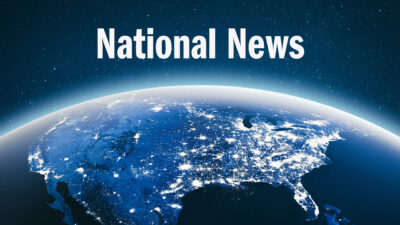
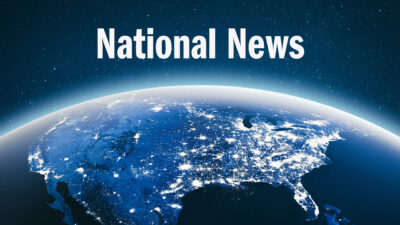
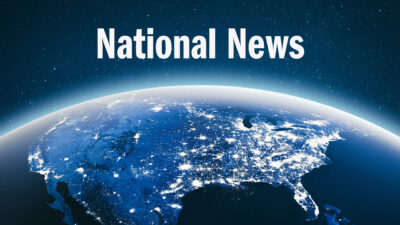
Senate heads toward final vote for ‘Big, Beautiful Bill’
June 30, 2025
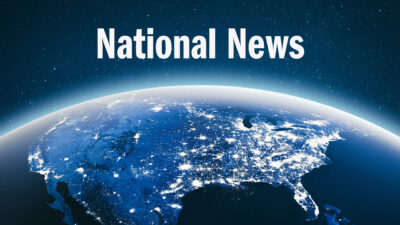
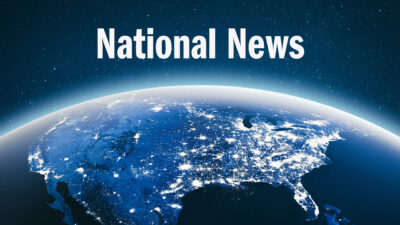
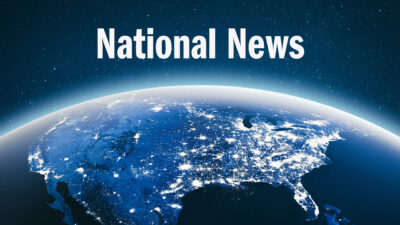
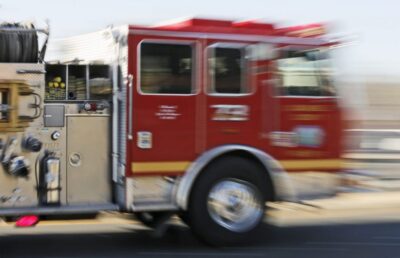
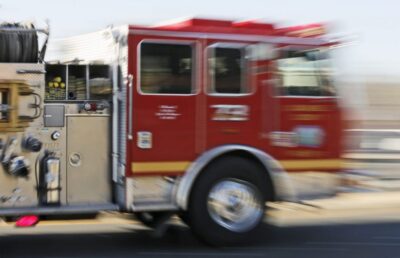
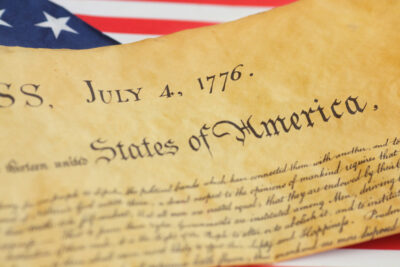
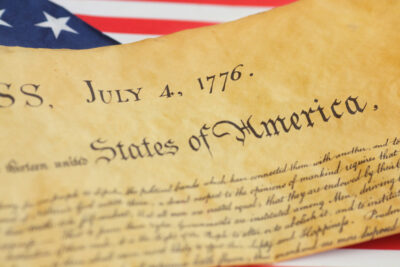
Why We Celebrate the Fourth of July
June 29, 2025